In-process monitoring is the key to 3D printing quality parts and to production that scales into true additive manufacturing (AM). While thermal imaging is the most obvious way to monitor certain types of 3D printing—such as laser powder bed fusion (LPBF)—acoustic monitoring is beginning to show promise, as well.
Content
Roland Loge, head of the Laboratory of Thermomechanical Metallurgy at the École Polytechnique federal de Lausanne (EPFL), says his team’s research shows that acoustic monitoring is not only viable, but potentially superior to thermal imaging. As lead researcher Milad Hamidi NASA explained in a press release, “The synergy of synchrotron X-ray imaging with acoustic recording provides real-time insight into the LPBF process, facilitating the detection of defects that could jeopardize product integrity.”
In essence, the researchers combined operando X-ray imaging with acoustic emission measurements, using the Swiss Light Source for the former and an ultra-sensitive microphone positioned inside the build chamber for the latter. By using an adaptive filtering technique to process the acoustic signals, the researchers were able to connect defects with their acoustic signatures.
Melt Pool Monitoring
The interactions between lasers and powder beds inside metal 3D printers are incredibly complex, with the melt pool fluctuating between solid, liquid and vapor phases. As a result, variables such as the angle of the laser or the geometry of the metal powder can sometimes lead to inter-regime instabilities within the melt pool, in which it shifts between conduction and keyhole regimes. It’s the latter type, specifically an unstable keyhole regime, that leads to porosity.
The EPFL researchers found an increment in the acoustic signal amplitude when the regime changed from conduction to keyhole, which they correlated with a change in laser absorptivity. By correlating between the acoustic signals and the x-ray images, the researchers were able to design a filtering algorithm that can predict melting regimes with a time resolution of 100 µs.
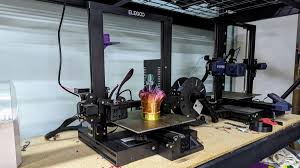
What’s particularly notable about this approach is that it obviates the need for machine learning and other computationally expensive forms of artificial intelligence (AI). All that’s needed is a simple filtering algorithm.
“This research paves the way for a better understanding and refinement of the manufacturing process, and will ultimately lead to higher product reliability in the long term,” said Logé.
The research is published under the title “Harmonizing sound and light: X-ray imaging unveils acoustic signatures of stochastic inter-regime instabilities during laser melting” in Nature Communications.
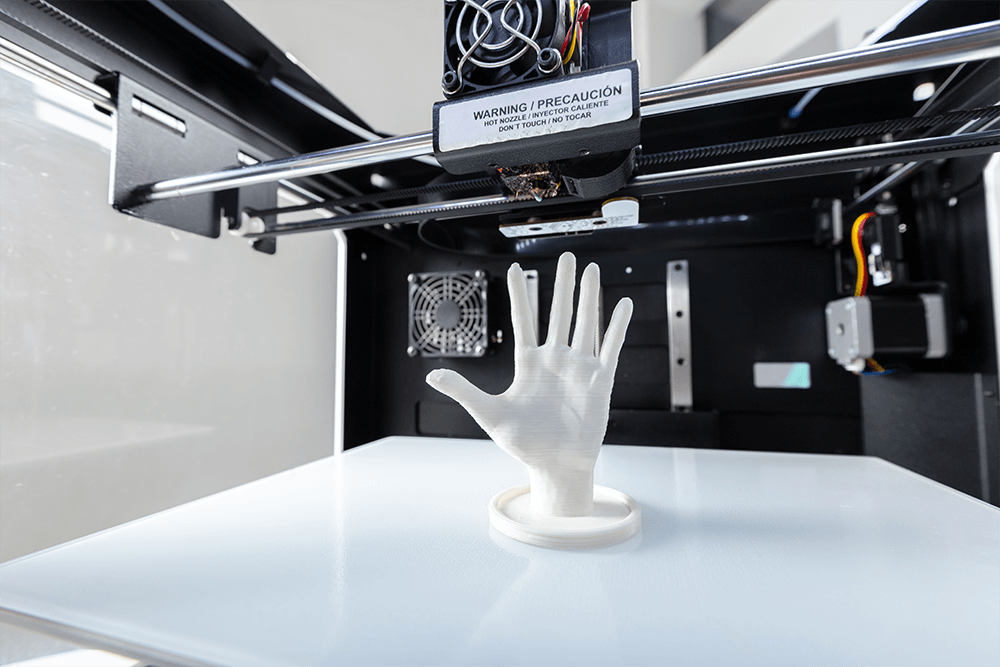
Add a Comment